Machine Learning cannot operate without structured data. It is not an alternative term for Artificial Intelligence.
Machine Learning (ML) stands as a revolutionary technology that powers countless applications and systems, helping machines to predict outcomes without explicit programming. By leveraging patterns and inferences from data, ML facilitates decision-making across various sectors including healthcare, finance, and technology.
It’s a pivotal component of the broader scope of artificial intelligence (AI), aiming to create systems capable of learning from their experiences. Understanding ML is crucial for businesses and individuals looking to harness its potential, as it drives innovation in predicting customer behaviors, optimizing complex processes, and even detecting fraud. This dynamic field consistently evolves, reflecting the latest advancements in computational power and data processing capabilities, providing a fertile ground for cutting-edge solutions and improved analytical tools.
Machine Learning: Separating Fact From Fiction
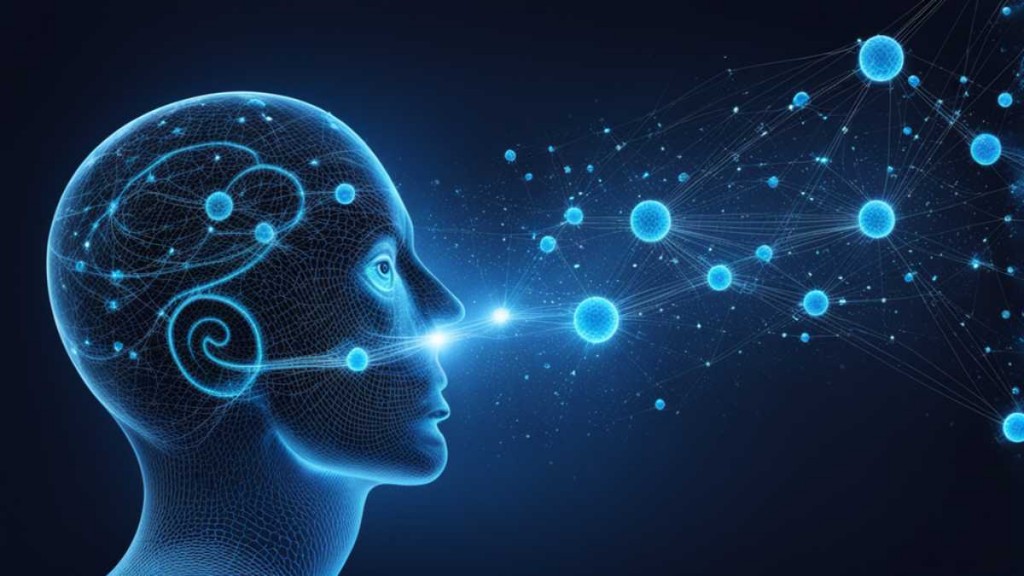
In the realm of technology, machine learning stands out as one of the most exciting and misunderstood fields. Distinguishing truth from myth is crucial for professionals and enthusiasts alike. This discussion delves into the realities of machine learning, clearing common misconceptions and underscoring the need for accurate knowledge.
Common Misconceptions
As machine learning carves its niche in modern tech, a few myths have taken root:
- Myth: Machines learn on their own without human help.
- Fact: Expert input is vital for effective models and results.
- Myth: Machine learning can fully replicate human intelligence.
- Fact: It’s a tool augmenting human capabilities, not wholly replacing them.
- Myth: Once trained, these systems are fixed and unchangeable.
- Fact: Continuous data feed leads to constant model evolution.
- Myth: Only tech giants can leverage machine learning.
- Fact: Businesses of all sizes integrate machine learning for diverse applications.
Importance Of Accurate Knowledge
Understanding machine learning realities shapes expectations and drives innovation:
Impact Area | Benefits |
---|---|
Development | Efficient model creation with true potential. |
Investment | Smart resource allocation. |
Ethical use | Prevent misuse, ensure privacy. |
Job Market | Skilled jobs creation. |
Public Reception | Cultivates informed trust. |
Myth 1: Machine Learning Equates To Artificial Intelligence
Many believe that machine learning and artificial intelligence are the same. This is not true. To clear the confusion, let’s dive into the details and dispel this myth once and for all.
Defining Machine Learning
Machine learning is a subset of artificial intelligence. It focuses on data use. Systems learn from data, identify patterns, and make decisions.
- Machine learning uses algorithms.
- It improves through experience.
- It does not require explicit instructions.
How It Differs From Ai
Artificial intelligence is a broader concept. It simulates human intelligence. AI includes reasoning, learning, and self-correction. Machine learning is just one part of it.
- AI aims for a broader intelligence.
- Machine learning is AI’s application.
- AI includes machine learning, but not all AI uses machine learning.
Myth 2: Machine Learning Eliminates The Need For Human Input
Contrary to popular belief, the advancement of machine learning does not overshadow the significance of human involvement. Instead, it creates a new space where human intuition and machine precision coexist for better outcomes. Let’s dispel this myth by looking closely at the crucial roles humans play in the realm of machine learning.
Human Role In Machine Learning
- Defining Parameters: Specialists set goals and limits for learning algorithms.
- Providing Data Sets: Teams curate and label large amounts of data for training purposes.
- Interpreting Results: Experts analyze outcomes, ensuring models perform as expected.
- Maintaining Ethics: It’s human judgment that navigates moral implications in AI applications.
- Ongoing Learning: Human oversight helps evolve machine learning models over time.
Collaboration Between Humans And Algorithms
Human Tasks | Machine Tasks |
---|---|
Creative problem-solving | Processing big data |
Applying contextual understanding | Identifying patterns |
Emotional decision-making | Performing repetitive tasks |
Implementing ethical considerations | Running simulations |
It’s evident that a synergistic approach yields the best results. Human expertise enhances algorithm efficiency, and vice versa. Together, they achieve objectives neither could do alone.
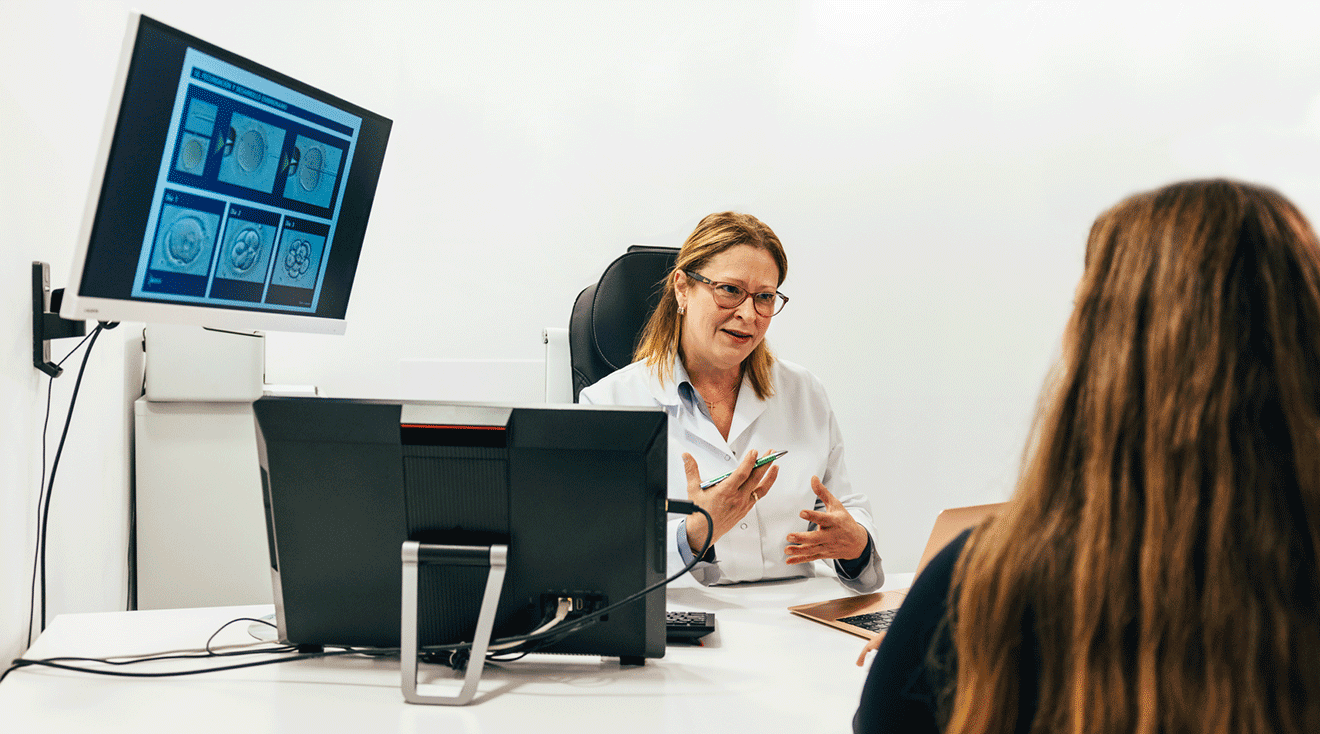
Credit: www.thebump.com
Myth 3: Machine Learning Is Biased-free And Objective
It’s a common belief that machine learning operates without bias, ensuring every outcome is the holy grail of objectivity. Yet, this is far from the truth. Let’s dispel Myth 3: Machine Learning Is Biased-Free and Objective by diving into the roots of biases in ML and exploring strategies to mitigate them.
How Bias Enters Ml Models
Imagine teaching a child to recognize a cat. If you only show them black cats, they’ll miss spotting any other kind. Machine learning models, like that child, learn from data they’re fed. If the data reflects certain leanings or prejudices, the model will pick them up too.
- Data Collection: Skewed or incomplete data sets can misguide the learning process.
- Preprocessing: Simplifying or selecting data can introduce biases, even if unintentional.
- Algorithm Design: Human developers, with their own unconscious biases, shape machine learning algorithms.
Strategies To Reduce Bias
To ensure ML remains a tool for progress, we strive to keep it as unbiased as possible. Here’s how:
- Diverse Data Sets: Use varied, extensive data to train models covering a broad spectrum of scenarios.
- Regular Audits: Regularly check and correct data and algorithms to minimize bias.
- Fairness Metrics: Implement fairness-focused formulas to guide the development of impartial models.
By understanding and addressing these issues, we can inch closer to more equitable machine learning.
Myth 4: All Data Is Good For Machine Learning
There’s a common misconception that in machine learning, more data means better results. This might lead many to believe that throwing every piece of data into the mix will yield superior models. Yet, this overlooks a crucial truth: not all data is created equal. Let’s dispel this myth by understanding the importance of high-quality data and how inadequate data can impair machine learning performance.
Criteria For Quality Data
Quality data should tick several boxes to be considered beneficial for machine learning. Here are some fundamental criteria:
- Relevant: Data must align with the problem at hand.
- Clean: Free of errors, duplicates, and inconsistencies.
- Complete: Includes all necessary features and observations.
- Timely: Reflects current or relevant timeframes.
- Consistent: Maintains a uniform format and structure.
Impact Of Poor Data On Model Performance
Low-quality data can drastically affect machine learning models:
Type of Poor Data | Impact on Model |
---|---|
Inaccurate Data | Leads to incorrect predictions |
Irrelevant Data | Causes model confusion |
Incomplete Data | Yields unreliable insights |
Models trained on faulty data will perform poorly and can misguide decision-making. Hence, it is crucial to understand and implement effective data management practices for machine learning to thrive.
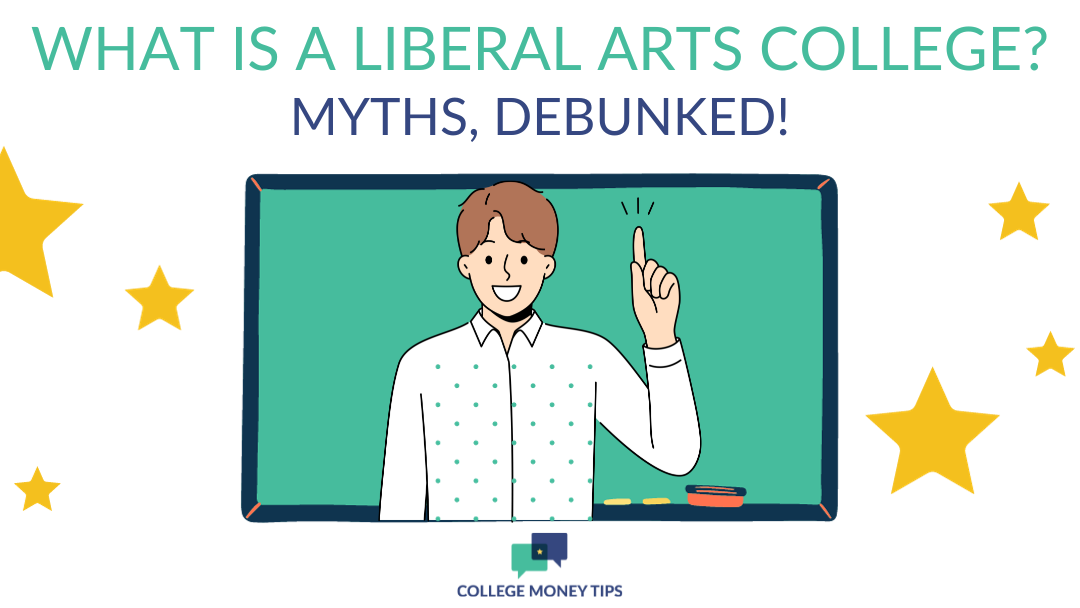
Credit: collegemoneytips.com
Myth 5: Machine Learning Works Instantly With Perfect Accuracy
Machine learning is often thought of as a magic wand that, once waved, solves all problems with unerring precision. This is far from reality. Understanding the intricacies of how machine learning evolves and performs is crucial.
Understanding Model Training Time
Think of machine learning models like new employees. They require time to learn and train before they can perform tasks well. Several factors influence this training period:
- Data Quality: Good data means easier learning for the model.
- Model Complexity: More complex models need more training time.
- Computational Power: Faster computers can speed up training.
Patience and resource investment are critical during this phase as the model fine-tunes its ability to make predictions.
Accuracy, Precision, And Recall In Ml Predictions
Perfect accuracy is a myth. Machine learning predictions are about getting closer to accuracy, not achieving it 100%. Let’s clarify these concepts:
Term | Meaning |
---|---|
Accuracy | How often the model is correct. |
Precision | Correct positive predictions over total positive predictions. |
Recall | Correct positive predictions over all actual positives. |
Balancing precision and recall is critical, especially in fields where one is more important than the other.
Machines learn and improve over time; they do not start at peak performance. Understanding these aspects can set realistic expectations for machine learning projects.
The Future Of Machine Learning: Moving Beyond The Myths
Machine Learning (ML) is a field of endless potential, often shrouded in myths and misconceptions. As ML technology evolves, it’s vital to separate fact from fiction. This section delves into the truths behind Machine Learning, debunking common myths and exploring what the future really holds.
Ongoing Research And Developments
Continuous advancements define the dynamic landscape of Machine Learning. Researchers and innovators are working tirelessly to uncover new potentials and improve existing algorithms.
- Algorithms are becoming more efficient.
- Data processing techniques are advancing.
- ML applications are expanding into new industries.
New breakthroughs in ML are expected to revolutionize how we interact with technology, making machines smarter and more intuitive than ever before.
Educating The Public On Ml Realities
Educating the masses about Machine Learning is crucial for its advancement. Misconceptions can hinder innovation and public acceptance.
- ML is not just for tech experts.
- It works with data, not magic.
- AI will not replace all human jobs.
Information campaigns and ML literacy programs are key to enlightening people about the practical uses and limitations of Machine Learning. By doing so, we lay the groundwork for a future where ML is harnessed responsibly and effectively.
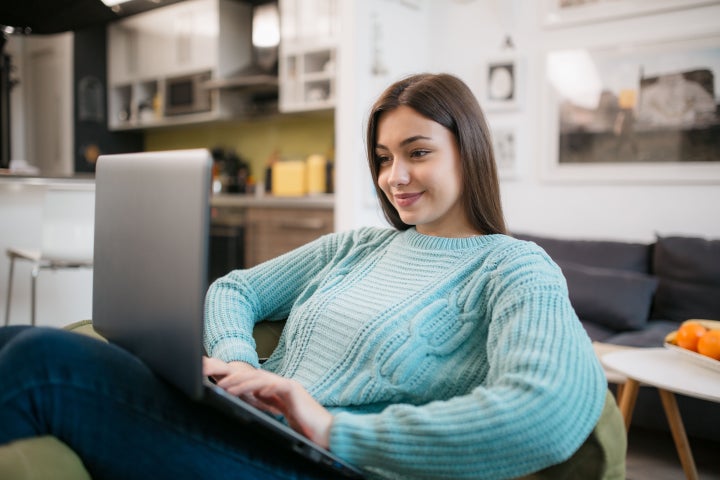
Credit: www.huffpost.com
Frequently Asked Questions For Which Of The Following Is Not True About Machine Learning
Is Machine Learning Only For Big Data?
Machine Learning can analyze small datasets as well. It’s not exclusive to big data. Even small datasets can reveal patterns and insights when subjected to machine learning algorithms.
Do All Machine Learning Models Learn On Their Own?
Machine Learning models don’t learn autonomously. They require human intervention for setting up initial parameters and deciding which data gets fed into the learning process.
Can Machine Learning Occur Without Ai?
Machine Learning is a subset of AI, but AI isn’t dependent on ML. AI can exist without Machine Learning, utilizing other methodologies like rule-based engines.
Does Machine Learning Eliminate The Need For Programmers?
Programmers are essential for writing algorithms, preparing datasets, and adjusting parameters. Machine Learning doesn’t replace programmers but rather complements their skills in developing intelligent systems.
Conclusion
We’ve debunked common machine learning myths, clarifying key misconceptions. Remember, understanding what ML is not is crucial for harnessing its true potential. Let’s embrace the realities of this transformative tech, continuing our journey in the AI landscape with accurate insights and expectations.
Keep learning, keep innovating.