Predictive maintenance in machine learning anticipates equipment failures using data analysis tools. It enhances operational efficiency by preventing downtime.
Predictive maintenance (PdM) techniques, powered by machine learning algorithms, are revolutionizing the field of equipment maintenance. By analyzing data from sensors and maintenance logs, these systems forecast when machinery will require servicing. This proactive approach enables businesses to perform maintenance only when necessary, reducing both downtime and costs associated with unplanned outages.
Machine learning models can identify patterns and anomalies that human analysts might overlook, making it possible to predict failures before they occur. As such, PdM serves as a critical component for any enterprise aiming to optimize its operations, improve asset longevity, and establish a smarter workflow. By leveraging machine learning, companies can transform their maintenance strategies, moving from reactive to predictive, and thereby gain a significant competitive advantage.
The Rise Of Predictive Maintenance
Predictive maintenance transforms how industries manage equipment. Unlike traditional methods of waiting for machines to break, it forecasts failures before they happen. This proactive approach uses machine learning to analyze data, increasing operational uptime and saving money. Let’s dive into this game-changing shift.
Shift From Reactive To Proactive Maintenance
Industries are moving from a fix-after-failure mode to a data-driven strategy.
- Machine learning algorithms study patterns in data.
- They predict problems before they worsen.
- This shift means fewer unexpected breakdowns.
Intelligent analysis optimizes maintenance schedules and extends machinery life.
Benefits For Industrial Uptime
Predictive maintenance is not just about avoiding repairs; it directly boosts uptime.
Benefit | Impact |
---|---|
Better resource allocation | Focus on critical areas, save time and labor |
Decreased downtime | Keep machines running, increase productivity |
Enhanced safety | Minimize accidents due to equipment failure |
Eco-friendly operations | Prevent waste of materials, energy-efficient |
Organizations optimize workflows and streamline operations. These cumulative advantages lead to significant cost savings and a stronger bottom line.
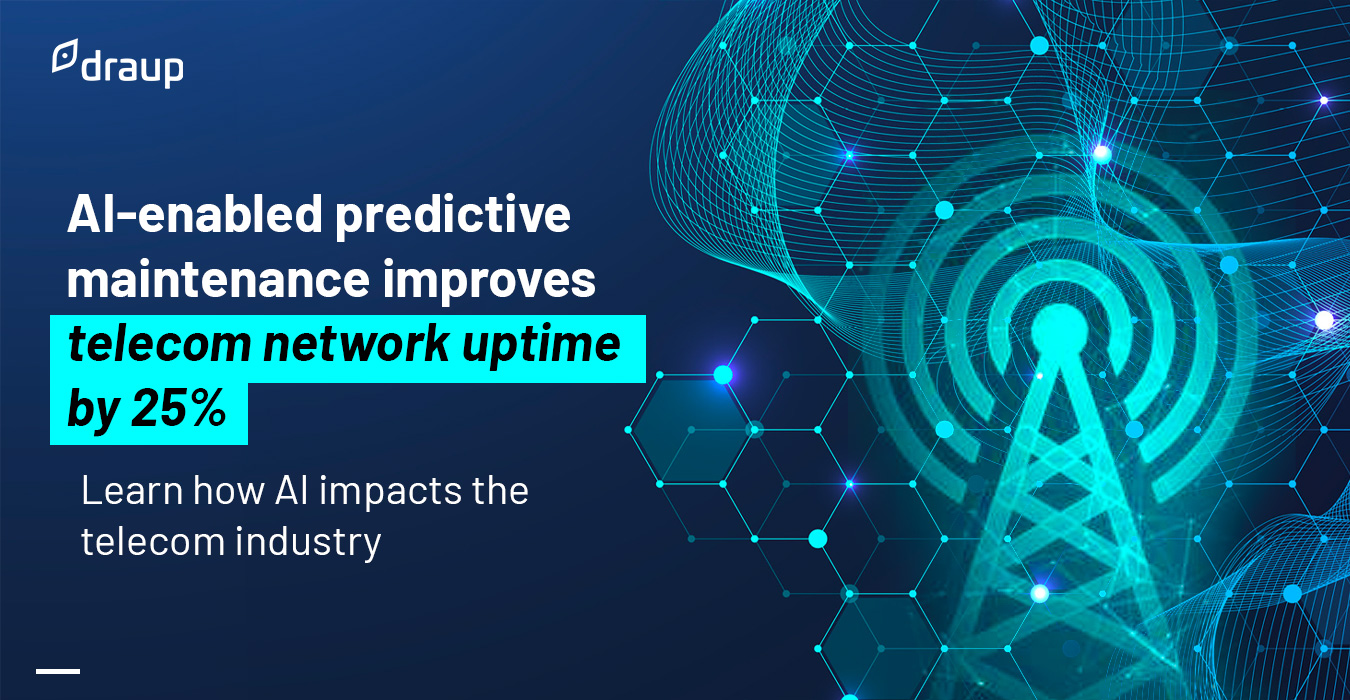
Credit: draup.com
Machine Learning At The Core
Machine Learning at the Core transforms how industries manage and maintain equipment. By predicting failures before they occur, businesses can save time and money. In this section, we explore the essentials of machine learning.
Basics Of Machine Learning For Maintenance
Machine learning is a form of artificial intelligence. It helps computers learn from data. In maintenance, machine learning predicts equipment issues before they happen. This helps companies avoid unexpected breakdowns.
Predictive maintenance relies heavily on data. Sensors on machines gather this data. Machine learning algorithms then analyze it. They learn from past patterns to forecast future performance.
- Data Collection: Sensors collect data such as temperature, vibration, and power usage.
- Data Processing: This data is cleaned and prepped for analysis.
- Model Training: Machine learning models use historical data to learn failure patterns.
- Prediction: The trained models then predict future breakdowns, allowing for timely maintenance.
Algorithms That Power Predictive Models
Different machine learning algorithms suit different maintenance needs. Each algorithm processes data to predict machinery health.
Algorithm Name | Use Case |
---|---|
Regression Analysis | Mimics the human brain to make complex predictions. |
Classification | Categorizes equipment states. |
Clustering | Finds data patterns without labels. |
Neural Networks | Mimics human brain to make complex predictions. |
Machine learning models become smarter over time. They continually learn from new data. This makes predictive maintenance accurate and reliable.
Data: The Lifeblood Of Prediction
In the realm of Predictive Maintenance, data acts as the essential force behind all reliable forecasts. Machine Learning (ML) thrives on data to identify patterns and preempt potential failures. This process ensures that machinery operates smoothly, minimizing downtime and preventing costly breakdowns.
Types Of Data For Machine Learning
Effective machine learning models depend on diverse data:
- Historical Data: Past information on machine performance and breakdowns.
- Real-time Data: Current data from sensors monitoring machinery.
- Environmental Data: External factors that might affect machine functionality.
Combining these data types helps ML algorithms predict equipment failures accurately.
Gathering And Preprocessing Maintenance Data
The process of refining raw data into a format suitable for ML is critical:
- Data Collection: Sensors and logs capture machine status and activities.
- Data Cleaning: This step involves removing errors or irrelevant data.
- Data Transformation: Data is structured into a format ready for analysis.
Preprocessed data is key for predictive algorithms to function optimally.

Credit: www.mouser.com
Implementing Predictive Maintenance
Implementing Predictive Maintenance with machine learning is transforming the way businesses manage their equipment. This tech predicts when a machine might fail. This means companies can fix things before they break. It saves money and time. Let’s look at how to make it work.
Steps To Deploy Machine Learning Models
- Collect data: Gather machine info like temperature and vibration.
- Choose algorithms: Pick the right machine-learning tools for your data.
- Train the model: Feed the data to the model so it can learn patterns.
- Test the model: Check if the model can predict problems correctly.
- Deploy the model: Put the trained model into the real world.
- Monitor performance: Keep an eye on the model to make sure it’s working.
Integrating With Existing Systems
To make predictive maintenance work, your machine learning models must talk to your current systems. This means:
- Creating data links between your machines and the new system.
- Ensuring the data is clean and in the right format for analysis.
- Setting up a UI for users to see the insights easily.
This way, your team can keep using their tools while still getting new, helpful info.
Real-world Applications
Machine learning is revolutionizing how we perform maintenance. By predicting when machines might fail, businesses save time and money. Let’s explore how predictive maintenance machine learning is applied in the real world.
Case Studies: Success Stories
Predictive maintenance has many success stories. Here are a few:
- Airlines – By predicting engine issues, airlines prevent delays and save on repairs.
- Manufacturing plants – Sensors predict equipment failure, reducing downtime and production losses.
- Energy sector – Power plants use machine learning to forecast turbine maintenance, ensuring uninterrupted power supply.
Industries Transformed By Predictive Maintenance
Many industries have transformed their maintenance strategies with machine learning. Here’s how:
Industry | Improvement |
---|---|
Transportation | Fewer vehicle breakdowns, optimized repair schedules. |
Healthcare | Medical equipment reliability increased, ensuring patient safety. |
Utilities | Minimized outages with preemptive maintenance on grids. |
Challenges And Considerations
Predictive maintenance machine learning is transforming industry practices. Yet, it’s not without hurdles. This section unpacks common challenges and considerations that must be addressed.
Obstacles In Applying Machine Learning
Integrating machine learning into predictive maintenance routines comes with obstacles:
- Data quality and volume: High-quality, abundant data are required.
- Model complexity: Models can become intricate, demanding expert tuning.
- Integration woes: Merging new systems with old poses operational issues.
These factors mean careful planning and resources are essential to succeed.
Ethical And Privacy Concerns
Protecting information is critical in machine learning applications:
Concern | Detail |
---|---|
Data security | Prevent unauthorized access to sensitive machine data. |
User privacy | Ensure personal user data stays private and is handled responsibly. |
Ethical use | Machine learning outputs must be used fairly and without bias. |
Addressing these concerns is vital for trust and the ethical use of technology.
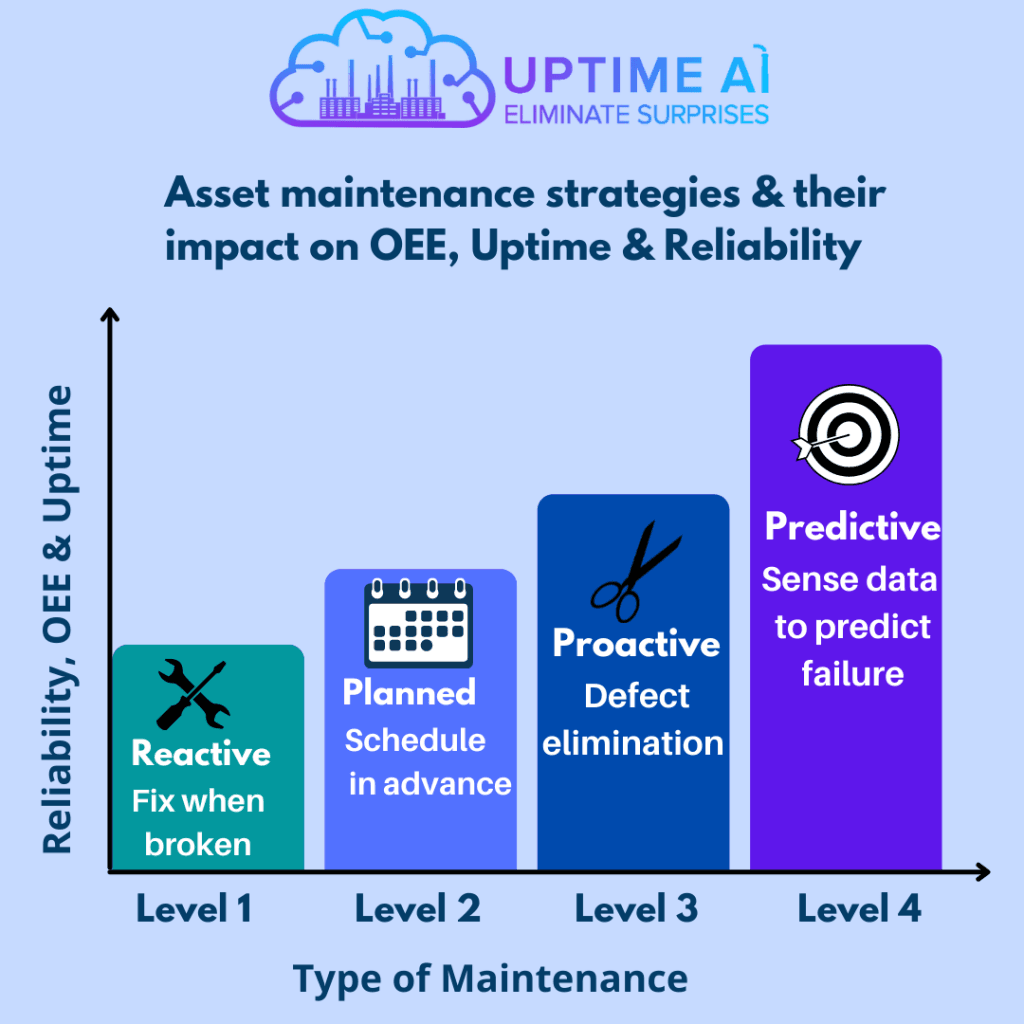
Credit: www.uptimeai.com
Frequently Asked Questions Of Predictive Maintenance Machine Learning
What Is Predictive Maintenance In Machine Learning?
Predictive maintenance utilizes machine learning to anticipate equipment failure before it happens. By analyzing data from machinery, algorithms identify possible issues that could lead to downtime, enabling proactive repairs and reducing maintenance costs.
How Does Machine Learning Improve Maintenance?
Machine learning enhances maintenance by processing large datasets to find patterns predicting failures. It streamlines maintenance schedules, extends equipment life, and optimizes repair tasks, thus significantly cutting unexpected breakdowns and associated expenses.
What Data Is Used For Predictive Maintenance?
Data for predictive maintenance includes machine usage statistics, historical maintenance records, sensor readings, and operational conditions. This data is crucial for training machine learning models to forecast potential equipment failures with higher accuracy.
What Are The Benefits Of Predictive Maintenance?
The benefits of predictive maintenance are immense, including reduced downtime, decreased maintenance costs, extended equipment lifespan, and improved safety. This proactive approach ensures timely interventions, avoiding costly emergency repairs and production losses.
Conclusion
Embracing predictive maintenance powered by machine learning is no longer futuristic—it’s a current necessity. Efficient operations hinge on anticipating equipment issues before they escalate. For businesses aiming to stay ahead, investing in this smart technology ensures minimal downtime and maximized productivity.
As machines become increasingly intelligent, the question isn’t if you’ll adopt this trend, but when.