Transactions on Machine Learning Research (TMLR) is a reputable journal for scholarly machine learning research. It publishes high-impact articles with rigorous methodologies and significant contributions.
Machine learning is an ever-evolving field that sits at the intersection of computer science and statistics. The Transactions on Machine Learning Research makes a substantial impact by publishing articles that present new theories, algorithms, or comprehensive experiments. Researchers and professionals from both academia and industry turn to TMLR for the latest discoveries and trends in machine learning.
The journal ensures all published work meets stringent peer-review standards, emphasizing originality and practical relevance. By keeping articles concise and focused, TMLR facilitates swift dissemination of research findings to a broader audience. Engaging with TMLR offers valuable insights into cutting-edge methodologies and applications that drive advancements in machine learning.

Credit: www.analyticsinsight.net
The Advent Of Machine Learning
The journey of Machine Learning (ML) is a captivating saga of how technology has advanced. It’s a tale of transforming raw data into smart algorithms. Today, ML is reshaping industries, revolutionizing how we handle tasks and unlocking potentials we once only dreamed of.
Evolution From Data Analysis
The leap from simple data analysis to ML is immense. Early computations were manual, tedious, and error-prone. Then, machines began making sense of data on their own. This shift turned information into insights without human intervention.
Key developments in technology have fueled this evolution. Faster processors, improved storage, and innovative algorithms led the way. Now, ML models can identify patterns in massive datasets in seconds, a task unthinkable for humans alone.
Key Milestones In Machine Learning History
- 1950s: The term ‘Machine Learning‘ is coined. Early work begins.
- 1960s: First neural networks developed. They mimic brain activity.
- 1980s: Decision trees and practical applications emerge.
- The 1990s: Support vector machines were introduced. Significant algorithm advancements.
- 2000s: Big data sparks a new wave of ML developments.
- 2010s: Deep learning revolutionizes ML fields.
Each milestone represents a leap forward. These moments have crafted the powerful ML tools we use today.
Core Concepts In Machine Learning
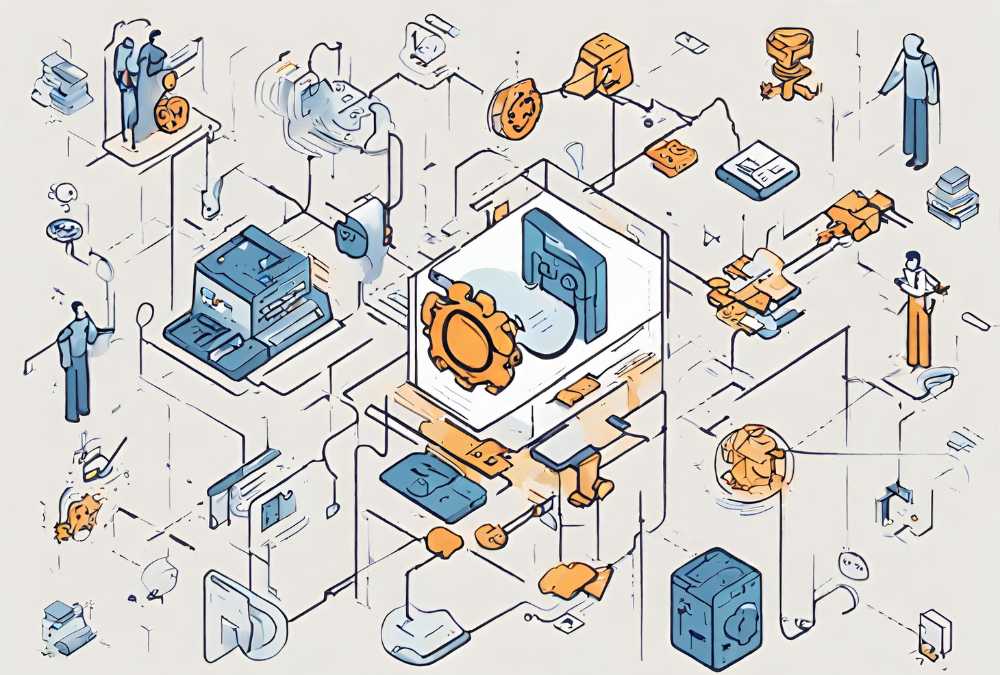
The world of Machine Learning is vast and complex, yet at its core, certain concepts remain fundamental. Understanding these principles is essential for anyone interested in the field, whether a newbie or an expert. Let’s delve into the bedrock of machine learning and discuss supervised versus unsupervised learning followed by the significance of algorithms.
Supervised Vs Unsupervised Learning
Machine Learning thrives on data to learn and make decisions. Two primary learning types exist: supervised and unsupervised. Supervised Learning uses labeled data. Think of it like learning with a guide.
- Input: Labeled data
- Output: Predictions based on data
- Examples: Spam filters, weather forecasting
Unsupervised Learning, however, finds patterns with no explicit guidance.
- Input: Unlabeled data
- Output: Groupings or associations
- Examples: Market segmentation, gene clustering
Choosing the right approach depends on the problem and the data available.
The Importance Of Algorithms
Algorithms are the heart of Machine Learning, providing the rules and processes that enable learning from data. Why Algorithms Matter:
- They transform data into insights.
- Different algorithms suit different tasks and data types.
- Efficient algorithms can save time and reduce costs.
Mastering algorithms unlocks the power to tackle complex tasks and grow knowledge from data.
Transformative Machine Learning Research
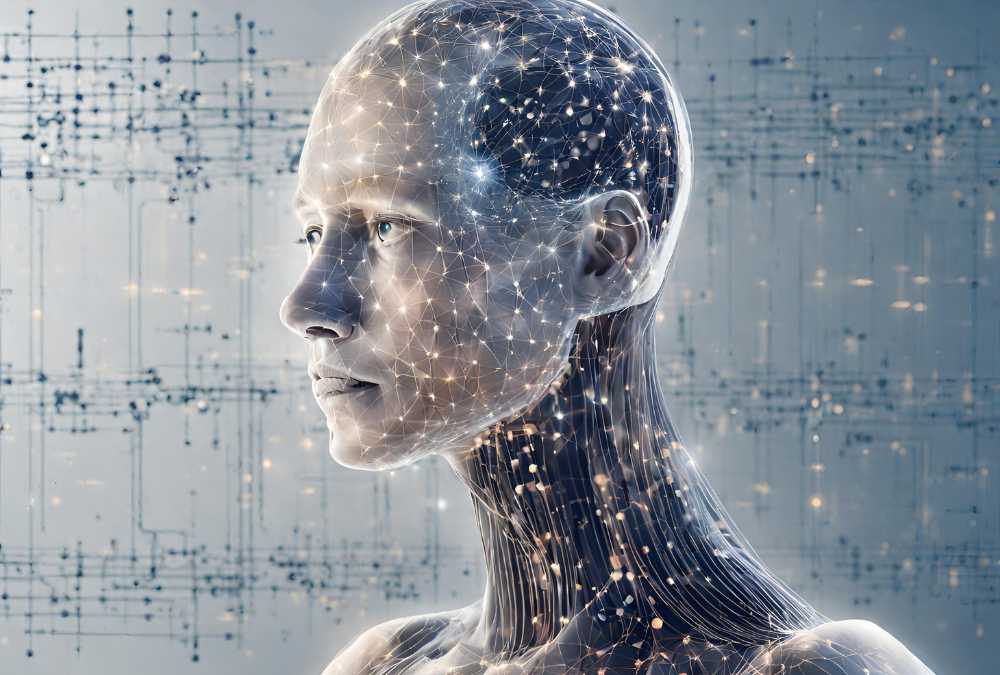
Transformative Machine Learning Research stretches the boundaries of what we thought possible with algorithms and data. Often characterized by novel approaches and significant advancements, this research paves the way for remarkable developments across various industries.
Breakthroughs Shaping The Field
The essence of innovation shines through in machine learning breakthroughs. These discoveries challenge old methods and introduce smarter, more efficient systems. Machine learning, a booming field, witnesses rapid progress with each passing year.
- Deep learning excels in image and speech recognition.
- Reinforcement learning drives advancements in robotics and gaming.
- Federated learning brings privacy improvements.
Case Studies Of Pioneering Work
Real-world applications demonstrate the power of transformative machine learning research. Let’s consider some groundbreaking case studies.
Year | Study | Impact |
---|---|---|
2016 | AlphaGo | Defeated world champion in Go, showcasing deep reinforcement learning. |
2018 | GANs in Art | Generated original artwork, blurring lines between AI and creativity. |
2020 | GPT-3 | Pushed the envelope in natural language processing and generation. |
Machine Learning In Application
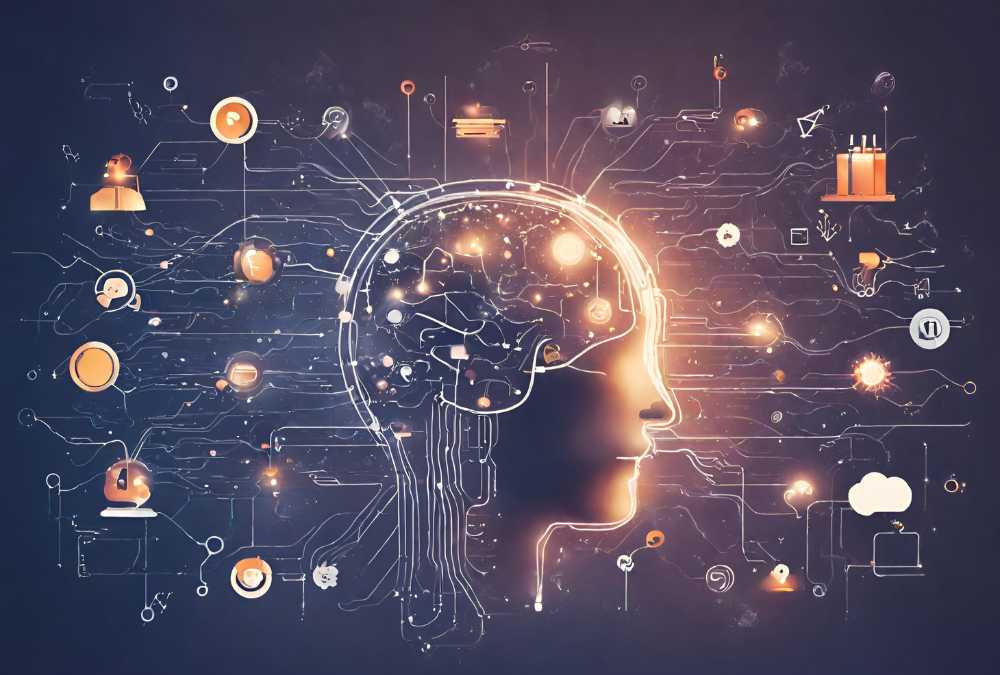
Imagine a world where machines learn like humans. That’s the magic of Machine Learning in Application. It’s like giving computers a brain to make decisions. This power is reshaping industries and changing our daily lives. Let’s explore how!
Industry-specific Use Cases
Every industry has its hero, and for many, machine learning wears the cape. Here are some ways it’s making a mark:
- Healthcare: Doctors use it to spot diseases early.
- Finance: Banks find fraud before it happens.
- Retail: Shops know what you want to buy next.
- Manufacturing: Factories prevent machines from breaking down.
In all these cases, data is the secret ingredient. Machine learning algorithms study this data to help industries improve and innovate.
Rise Of Ai In Everyday Life
Artificial Intelligence (AI) is not just a sci-fi fantasy; it’s our new reality. It’s in the home, at work, and everywhere in between. Let’s take a peek:
WhereHow Machine Learning Helps | |
---|---|
Home | Smart speakers play music and answer questions. |
Transport | GPS apps predict traffic and plan routes. |
Shopping | Online stores recommend products you’ll love. |
Entertainment | Streaming services know the shows you’ll binge-watch. |
The rise of AI makes life easier and more fun. It’s like having a personal assistant who learns to serve you better every day.
Challenges And Ethical Considerations
The realm of Machine Learning Research is not just about technological advancements but also about navigating through a maze of complex challenges and ethical dilemmas. It’s crucial to address these to ensure that technology benefits all sections of society while safeguarding individual rights.
Bias And Fairness
Eliminating bias is a steep challenge in Machine Learning. Algorithms learn from data, which may include historical biases. This can lead to unfair decisions against certain groups.
- Examining data sources for inherent prejudices
- Ensuring diversity in training data
- Implementing algorithmic audits for fairness
Data Privacy And Security
Protecting user data is paramount in machine learning applications. Breaches can lead to loss of sensitive information, harming individuals and organizations.
Aspect | Action |
---|---|
Data Encryption | Secure data in transit and at rest |
Access Controls | Ensure only authorized personnel handle data |
Anonymization Techniques | Remove identifiable information from datasets |
Regular security audits and compliances, such as GDPR, are essential. They maintain trust and integrity in machine learning ecosystems.
Future Of Machine Learning Research
The landscape of machine learning is ever-evolving, with new breakthroughs reshaping research directions. This continuous advancement promises exciting developments for the future of machine learning research. Anticipating future trends and breakthroughs is key to sustaining innovation and maintaining a competitive edge.
Predicting The Next Big Thing
To anticipate where machine learning is heading, analyzing current trends is crucial. Researchers and practitioners are striving to unveil emerging patterns that indicate the next big leap in ML capabilities. This could be a novel algorithm, a transformative model, or a groundbreaking application.
- Focus on unsupervised and reinforcement learning can potentially lead to more autonomous AI systems.
- The scalability of models will likely invite developments targeting resource efficiency and faster computation.
- Integration of quantum computing with ML suggests a transformative paradigm that can redefine data processing.
The Role Of Interdisciplinary Studies
The merge between machine learning and other disciplines is nurturing unparalleled innovation. Interdisciplinary studies provide fresh perspectives, unearthing novel applications and methodologies.
Field | Impact on ML |
---|---|
Neuroscience | Enhances neural network design mirroring human cognition. |
Quantum Physics | Paves the way for quantum algorithms that may revolutionize AI. |
Genetics | Boosts predictive analytics in genetic mutation and disease spread. |
Interdisciplinary studies also promote the development of ethical AI, handling bias, and transparency in machine learning systems.
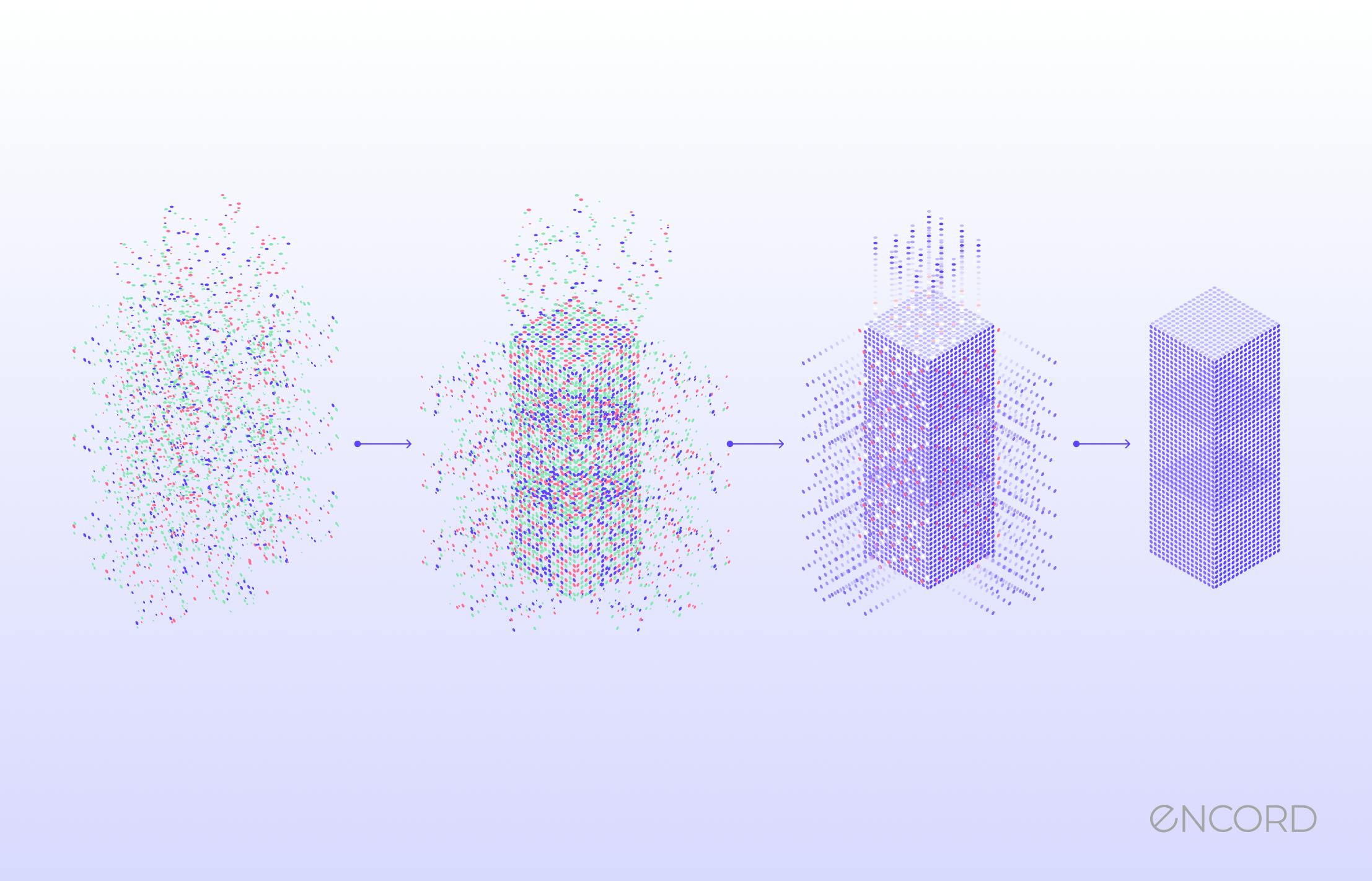
Credit: encord.com
Frequently Asked Questions For Transactions On Machine Learning Research
What Is Machine Learning Research?
Machine learning research is the scientific study of algorithms and statistical models. It enables systems to improve tasks through experience without using explicit instructions.
How Is Machine Learning Applied?
Machine learning is applied in various sectors like healthcare, finance, and technology. It improves processes by making data-driven predictions and decisions.
What Are The Types Of Machine Learning?
There are mainly three types: supervised, unsupervised, and reinforcement learning. Each type has different approaches for modeling and analyzing data.
Why Is Machine Learning Important?
Machine learning is important as it allows for more effective and efficient analysis of large data sets. It leads to innovative solutions across industries.
Conclusion
Navigating the evolving landscape of machine learning research is essential for any forward-thinking entity. We’ve delved into trends, techniques, and future trajectories, laying a clear path for understanding. Staying informed ensures you remain at the forefront of innovation. Embrace these insights to forge ahead in the dynamic world of machine learning.