Discover how Azure Machine Learning offers cost-effective solutions for your projects. Learn about pricing tiers and optimization.
Hey there! With machine learning becoming more and more essential for businesses today, you’ve probably been looking into solutions like Azure Machine Learning. And if you’re like most people, one of the first things on your mind is likely “How much is this gonna cost me?” Totally get it. You want to leverage the power of machine learning, but you need it to be affordable too.
Well, you’ve come to the right place! In this article, we’ll break down Azure Machine Learning’s pricing tiers and show you how to optimize costs for your specific needs. We’ll look at things like compute instances, data storage, and more. Our goal is to help you find the most cost-effective options so you can reap the benefits of machine learning without breaking the bank. Sound useful? Read on to get the inside scoop!
Overview of Azure Machine Learning and Its Benefits
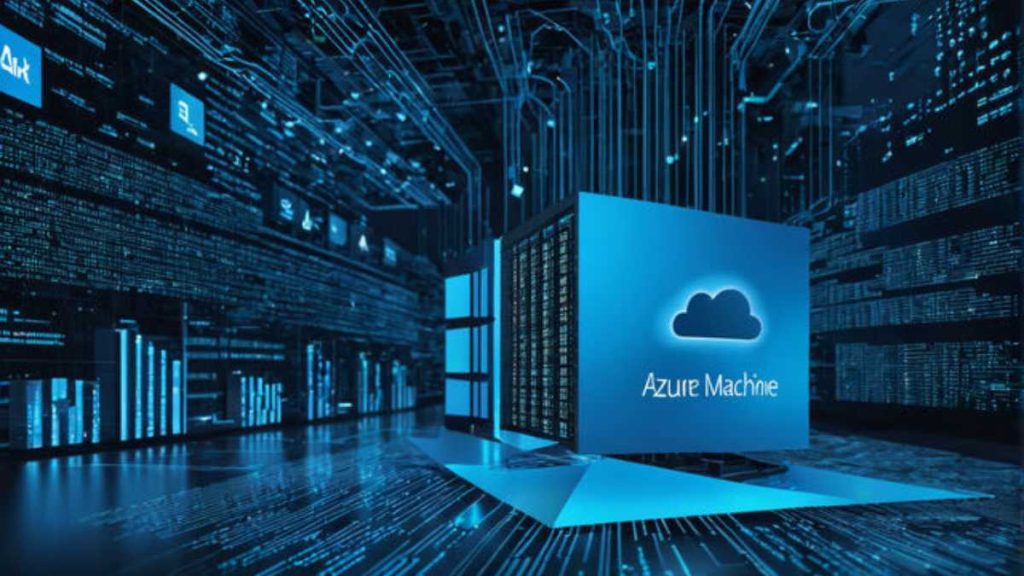
Feature | Description | Benefits |
---|---|---|
Cloud-Based Platform | Azure Machine Learning is a Microsoft cloud service providing tools and resources for machine learning development and deployment. | Accessibility: Work from anywhere with internet access. Scalability: Flexible compute power to match project needs. Cost-effectiveness: Pay for what you use. |
End-to-End Workflow | Covers all stages of the machine learning process: | Efficiency: Streamlined process from start to finish. Collaboration: Easy sharing and teamwork across the project. |
Data Preparation & Experimentation | Tools for data loading, cleaning, transformation, feature engineering, and model experimentation. | Improved Data Quality: Ensure data is suitable for modeling. Faster Experimentation: Quickly test different modeling approaches. |
Diverse Model Training Algorithms | Supports supervised, unsupervised learning, and reinforcement learning. Offers pre-built algorithms and the flexibility to customize your own. | Algorithm Choice: Find the right fit for your problem. Optimization: Achieve high model performance. |
Automated Machine Learning (AutoML) | Simplifies model selection and hyperparameter tuning. | Efficiency: Saves time for data scientists. Accessibility: Makes ML accessible to non-experts. |
MLOps Integration | Provides tools for model deployment, monitoring, retraining, and management in production. | Reliability: Ensure models perform well in real-world use. Agility: Adapt and update models quickly. Governance: Manage models responsibly. |
Integration with Azure Ecosystem | Works seamlessly with other Azure services like Azure Data Lake Storage, Azure Databricks, Azure Synapse, etc. | |
Open-Source Compatibility | Supports popular libraries and frameworks like TensorFlow, PyTorch, scikit-learn, etc. |
Low-Cost Options
Azure Machine Learning offers flexible pricing so you can choose the tier that fits your needs and budget. The free tier gives you access to the studio interface and enough computing power to build and deploy models. When you’re ready to scale up, pay-as-you-go options provide on-demand access to GPUs and fast CPUs.
Automated ML
The AutoML feature automatically tests hundreds of models to find the most accurate one for your data. Just upload your dataset, select the machine learning task, and AutoML will do the rest. It can save you valuable time and resources, especially if you’re new to machine learning.
Powerful Tools & Integrations
Azure ML provides all the tools you need to build, train, and deploy models at an enterprise scale. Use Python or the visual interface to clean data, engineer features, train models, and monitor performance. Integrate with open-source libraries like PyTorch, TensorFlow, and scikit-learn. Deploy models as web services, on IoT devices, or directly into applications.
Robust Security & Governance
With role-based access control, you can grant users access to only the resources they need. Encrypt data at rest and in transit to meet compliance requirements. Track changes to experiments, datasets, and models for auditing purposes. These enterprise-grade features give you control and oversight over all your machine learning activities in Azure ML.
Whether you’re just getting started with machine learning or building models for mission-critical applications, Azure ML has you covered. With powerful tools, money-saving options, and robust security, you’ll have everything you need to make your machine-learning projects a success.
Azure Machine Learning Pricing Options
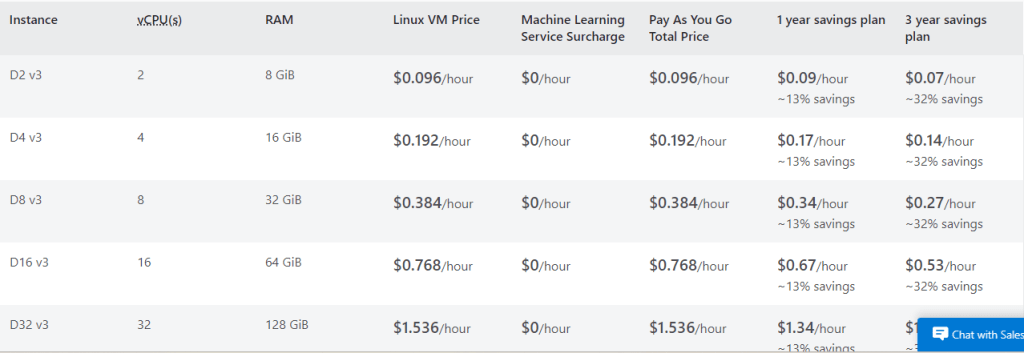
Azure Machine Learning offers three pricing tiers to suit your needs: Free, Basic, and Enterprise. Let’s explore what each tier offers.
Service/Resource | Pricing Model | Key Considerations |
---|---|---|
Compute Instances | Pay-as-you-go hourly rates based on virtual machine (VM) size and type. | Ideal for development and experimentation. |
Free Tier
The free tier is ideal if you’re just getting started with machine learning or want to build small models. You get access to the Azure ML studio to build, train, and deploy models, along with limited compute resources. The free tier is a great way to learn the ropes without spending a dime.
Basic Tier
The basic tier provides more robust capabilities for serious machine-learning projects. You get access to GPUs, increased storage, and higher compute limits. The basic tier starts at $50/month, so it’s very budget-friendly. This tier is perfect if you want to build and deploy production-ready models with more advanced algorithms and lots of data.
Enterprise Tier
The enterprise tier delivers the most powerful machine-learning tools and infrastructure. It offers specialized VM types with multiple GPUs, virtually unlimited storage, and high compute limits. You also get enterprise-level security and support. Pricing for the enterprise tier is customized based on your specific needs. This tier is ideal for large organizations running many complex machine-learning workloads.
With three tiers to choose from, Azure Machine Learning has a solution for machine learning projects of any scale. The pricing is very competitive, and you only pay for what you use, so you can start small and scale up as your needs grow. Azure Machine Learning delivers advanced ML tools at a cost that won’t break the bank.
Tips to Optimize Your Azure Machine Learning Costs
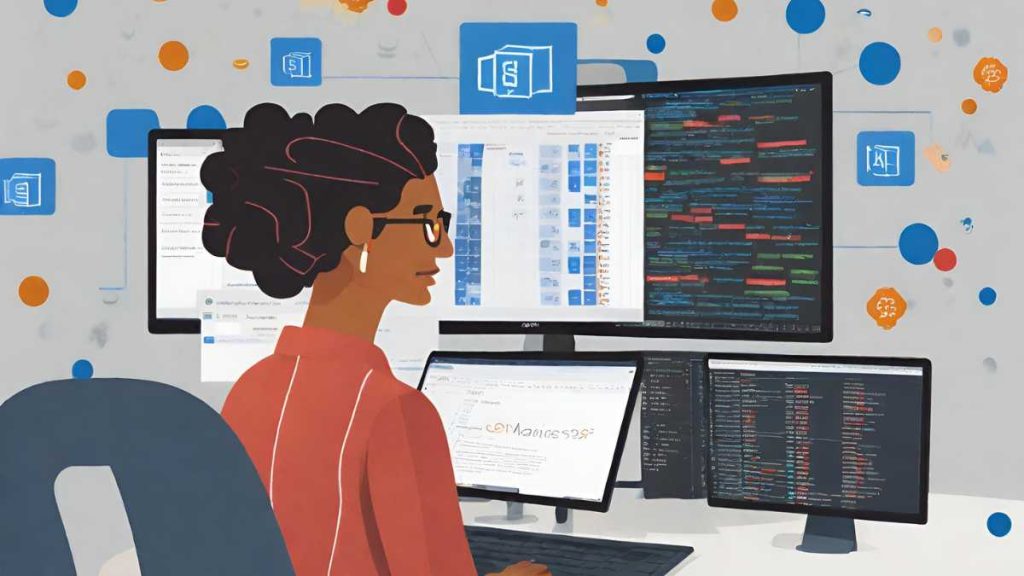
To get the most out of your Azure Machine Learning investments without breaking the bank, here are a few tips for optimizing costs:
Tip | Description | Considerations |
---|---|---|
Right-Size Compute | Match VM size/type to your workload. Avoid overprovisioning with excess CPU, memory, or GPUs. | * Start with smaller instances and monitor performance. Scale up if needed. |
Autoscaling for Clusters | * Best for consistent computing needs. | * Define minimum and maximum node counts. Set appropriate scaling thresholds. |
Low-Priority VMs | Use low-priority VMs for non-critical workloads. These offer significant discounts but may be preempted. | * Suitable for batch jobs or fault-tolerant training. |
Azure Reserved Instances | Commit to long-term VM usage (1 or 3 years) for potential cost savings. | * Best for consistent compute needs. |
Idle Shutdown | Enable automatic shutdown of compute instances when inactive for a set time. | * Ideal for development or testing environments. |
Scheduled Start/Stop | Schedule compute instances to run only during specific hours, saving costs outside of those times. | * Useful if usage patterns are predictable. |
Spot VMs | Take advantage of unused Azure capacity with spot VMs. Deep discounts, but instances can be preempted. | * Good for interruptible workloads. |
Choose the Right Region | Azure costs can vary between regions. Choose the most cost-effective region that meets your requirements. | * Consider data location and compliance needs. |
Cost Monitoring & Alerts | Set up Azure Cost Management and alerts to track spending and identify unexpected cost increases. | * Proactive management prevents surprises. |
Choose a Suitable Pricing Tier
Select a pricing tier that matches your needs. The Basic tier is free and good for learning, while Enterprise is ideal for large-scale production models. For most users, the Standard tier at $0.5/hour of computing will work great. You can also save by purchasing Azure Machine Learning units (MLUs) upfront.
Use Low-Priority VMs
For dev/test workloads, use ev3 or other low-priority VMs which can save up to 80% compared to pay-as-you-go VMs. Your jobs may take longer to start, but the cost savings are huge.
Automate and Scale Efficiently
In the real world, your models will need to handle huge amounts of data and scale efficiently. Automate as much as possible using the Azure CLI, SDKs, or Azure Resource Manager templates. This minimizes manual intervention and wasted computing time.
Choose the Right VM Size
Select an appropriately sized VM for your workloads. Don’t use an expensive VM with lots of power if you only need a small one. Monitor your VM usage and scale up or down as needed to optimize costs.
Delete Resources When Done
Remember to stop or delete compute resources like notebooks, datasets, and endpoints when you no longer need them. Leaving unused resources running can significantly increase your costs over time.
By following these tips, you’ll be able to build and deploy machine learning models on Azure without blowing your budget. Azure Machine Learning is designed to be cost-effective, so take advantage of all the options for optimizing your spend. Spend some time evaluating different options for your workloads—it can really pay off!
Comparing Azure ML to Other Cloud ML Services
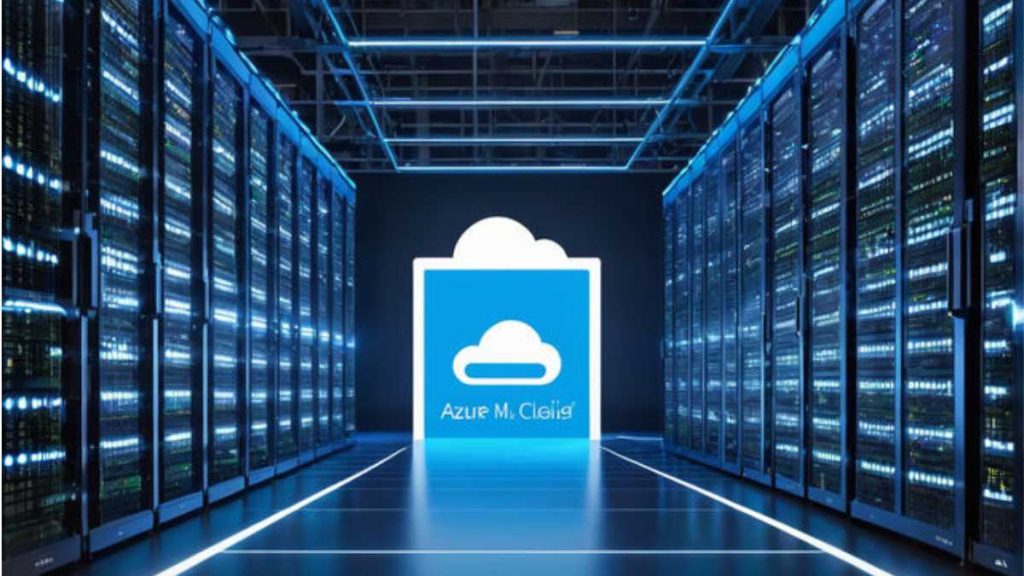
Feature | Azure ML | AWS SageMaker | Google Cloud AI Platform |
---|---|---|---|
Ease of Use | User-friendly interface with drag-and-drop components. Strong for both beginners and experts. | Vertex AI includes AutoML Tables, and AutoML for images, video, and text. | Simple for basic use, but customization and advanced features can be complex. |
Algorithm & Framework Support | Strong support for Google’s TensorFlow, and tight integration with other Google Cloud services. | Extensive algorithm selection. Integration with AWS-specific deep learning frameworks like MXNet. | Wide range of pre-built algorithms, supports popular open-source frameworks like TensorFlow, PyTorch, and sci-kit-learn. |
AutoML Capabilities | Azure Automated ML offers model selection, parameter tuning, and ease of use. | SageMaker Autopilot for end-to-end model building. | Pay-as-you-go for computing, storage, and managed services. Potential discounts with reserved instances. |
MLOps Features | Model deployment, management, and monitoring tools. CI/CD pipeline integration. | SageMaker Pipelines for managing end-to-end workflows. | Vertex AI Pipelines, feature store, model registry, and continuous monitoring. |
Integration with Cloud Ecosystem | Seamless integration with other Azure services (storage, data, analytics). | Works well with AWS services like S3, Redshift, etc. | Tight integration with Google Cloud Platform (BigQuery, Cloud Storage, Dataflow). |
Pricing | Pay-as-you-go model, pricing varies based on the services used. | Similar pricing, with pay-as-you-go for underlying resources. | Pay-as-you-go model, pricing varies based on services used. |
AWS SageMaker
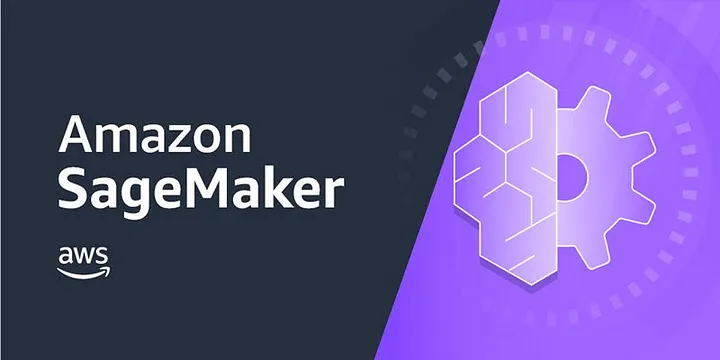
Like Azure ML, AWS SageMaker offers machine learning tools and infrastructure. SageMaker has a slightly steeper learning curve and requires more technical expertise to get started. It provides greater flexibility and control for experienced data scientists. However, for small and midsize businesses, Azure ML may be more accessible and affordable.
Google AI Platform
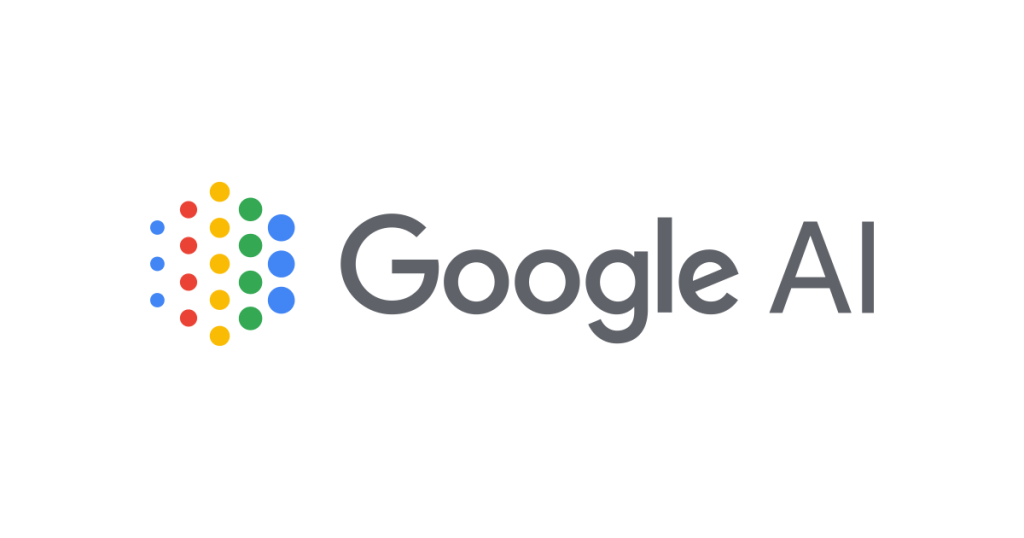
Google’s AI Platform is a managed service for building and deploying ML models. It offers many of the same features as Azure ML, including AutoML for automatically building models. However, Google’s AI Platform can be more expensive, especially if you’re not already using other Google Cloud services. Azure ML often represents the best value for businesses with limited ML expertise or budget.
IBM Watson
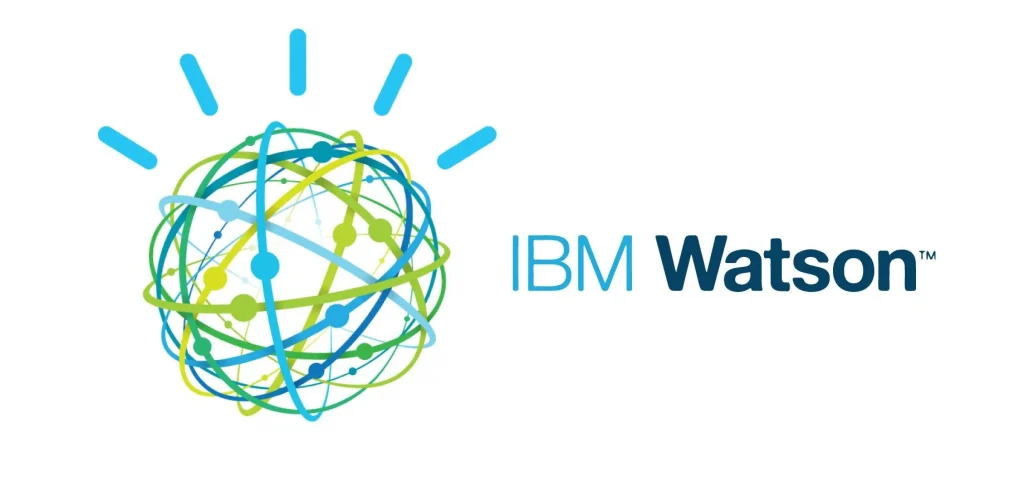
IBM’s Watson platform includes machine learning and AI services for tasks like natural language processing, computer vision, and predictive analytics. However, Watson can be significantly more expensive than Azure ML for many use cases. Watson also has a reputation for being more complex to set up and integrate. For most businesses, Azure ML will likely be the more practical and cost-effective choice.
In summary, while there are other reputable cloud ML services, Azure ML stands out for its combination of power, simplicity, and affordability. For small to midsize companies venturing into machine learning, Azure ML removes many of the technical and financial barriers that might otherwise hold you back from building innovative AI solutions. With Azure ML, you can start small and scale up as your needs and expertise grow over time.
Azure Machine Learning Price FAQs
How much does Azure Machine Learning cost?
Azure Machine Learning offers a pay-as-you-go pricing model, so you only pay for what you use. The service starts at $0.50 per hour for a D2v2 VM. The exact cost will depend on the machine types, compute instances, and storage you choose for your workflows and models.
Are there any free tiers available?
Yes, Azure Machine Learning has a free tier with access to the studio UI, SDK, and a limited number of compute resources to get started. The free tier includes 1 free D2v2 VM hour per month and 5GB of file storage. This is ideal for small proof-of-concept projects, but for full-scale enterprise machine learning, you’ll want to use the pay-as-you-go pricing.
How can I optimize costs?
There are a few ways to optimize your Azure ML spend:
- Choose less powerful VM types like D2v2 or D3v2 instead of more advanced machines for development and testing. Only use more powerful VMs for training complex models.
- Delete compute targets and compute instances when not in use. Any VM that is stopped will not incur charges.
- Use automation to start and stop environments on a schedule. This ensures machines are only running when needed for active workloads.
- Take advantage of Azure Hybrid Benefit for Windows Server to use your existing Windows Server licenses and save up to 40% on VM costs.
- Consider using Azure Spot VMs which can save up to 90% compared to pay-as-you-go prices for workloads that can handle interruptions.
- Use managed services like Azure Databricks and Azure Data Factory which provide serverless computing for running jobs and workflows.
- Choose a region with lower prices for computing like East US, West US 2, North Europe, or Southeast Asia. Pricing varies by region based on infrastructure costs.
By following these best practices, you can build cost-effective machine-learning solutions on Azure while still leveraging the full power of the platform. The key is choosing the right tools for the job and optimizing resources for maximum efficiency.
Conclusion
So there you have it. Azure Machine Learning provides flexible pricing options that can help you get the most bang for your buck. By understanding the pricing tiers, optimizing your setup, and taking advantage of credits and free tiers, you can build and deploy cost-effective ML solutions on Azure.
The key is choosing what makes sense for your specific needs. Start small, monitor spend, and scale up as your projects grow. With a bit of planning, you can do amazing things with machine learning on Azure without breaking the bank. The future is yours to shape with the power of AI – and Azure gives you the tools to build it affordably.